728x90
반응형
light BGM은 인코딩이 필요없음 !
결측치도 처리 안해도 됨 (오류나면 해주셈)
파라미터 튜닝은 max_depth =5 n_estimators=400, learning_rate=0.02
https://dataq.goorm.io/exam/116674/%EC%B2%B4%ED%97%98%ED%95%98%EA%B8%B0/quiz/2
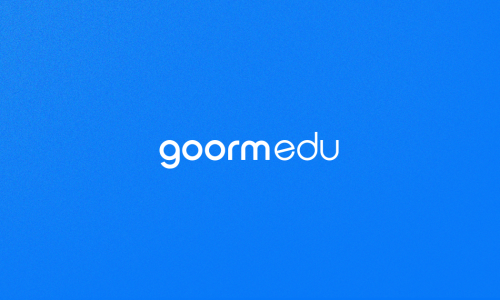
(체험) 제1유형 (풀이용) - 빅데이터분석기사 실기 체험
제공된 데이터(data/mtcars.csv)의 qsec 칼럼을 최소-최대 척도(Min-Max Scale)로 변환한 후 0.5보다 큰 값을 가지는 레코드 수를
【제출 형식】에 맞춰 답안 작성 페이지에 입력하시오.
【제출 형식】 ㉠정수(integer)로 입력 (단, 소수점을 포함한 경우 소수점 첫째 자리에서 반올림하여 계산) ㉡ 정수 답안만 입력
◾빅데이터분석기사 체험환경 가이드 링크
dataq.goorm.io
####### 분류 #######
import lightgbm as lgb
model = lgb.LGBMClassifier(random_state=0)
model.fit(X_train, y_train)
y_pred = model.predict(X_test)
# y_pred = model.predict_proba(X_test) #평가기준 roc-auc일 때
####### 회귀 #######
import lightgbm as lgb
model = lgb.LGBMRegressor(random_state=0)
model.fit(X_train, y_train)
y_pred = model.predict(X_test)
# 하이퍼파라미터 튜닝은 xgboost(작업형2 모의고사3 강의)과 동일하게 사용하면 됩니다.
import pandas as pd
X_test = pd.read_csv("data/X_test.csv")
X_train = pd.read_csv("data/X_train.csv")
y_train = pd.read_csv("data/y_train.csv")
train = pd.concat([X_train, y_train['gender']], axis=1)
test = X_test.copy()
# 탐색적 데이터 분석
print(train.shape)
print(train.info())
print(train.isnull().sum())
#결측치 처리
train['환불금액'] = train['환불금액'].fillna(0)
test['환불금액'] = test['환불금액'].fillna(0)
# 인코딩을 안하는 대신, category로 형변환을 해줘야한다 (object)
# 테스트 데이터도 같이 해줘야하는건 잊지마시오
train['주구매상품'] = train['주구매상품'].astype('category')
train['주구매지점'] = train['주구매지점'].astype('category')
test['주구매상품'] = test['주구매상품'].astype('category')
test['주구매지점'] = test['주구매지점'].astype('category')
print(train.info())
from sklearn.model_selection import train_test_split
X_train, X_val, y_train, y_val = train_test_split(train.drop('gender', axis=1), train['gender'], test_size=0.2, random_state=0)
print(X_train.shape, X_val.shape, y_train.shape, y_val.shape)
import lightgbm as lgb
model = lgb.LGBMClassifier(random_state=0, max_depth=5, n_estimators=400, learning_rate=0.02)
model.fit(X_train, y_train)
pred = model.predict_proba(X_val)
from sklearn.metrics import roc_auc_score
print(roc_auc_score(y_val, pred[:,1]))
pred = model.predict_proba(test)
pd.DataFrame({'pred':pred[:,1]}).to_csv('result.csv', index=False)
# print(pd.read_csv('result.csv'))
728x90
'IT&게임 > 빅데이터분석기사(빅분기)' 카테고리의 다른 글
빅분기 작업형2 기출 정리 (0) | 2024.06.18 |
---|---|
인코딩 정리(원핫인코딩/레이블인코딩) (0) | 2024.06.18 |
[빅분기] 여러가지 에러 케이스 (0) | 2024.06.18 |
빅데이터분석기사 제2유형 : 회귀문제 예제1 (0) | 2024.06.07 |
빅데이터분석기사 제2유형 : 분류문제 예제1 (0) | 2024.06.07 |
댓글